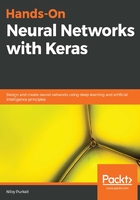
Understanding the role of the bias term
So, now we have a good idea of how data enters our perceptron; it is paired up with weights and reduced through a dot product, only to be compared to an activation threshold. Many of you may ask at this point, what if we wanted our threshold to adapt to different patterns in data? In other words, what if the boundaries of the activation function were not ideal to separately identify the specific patterns we want our model to learn? We need to be able to play with the form of our activation curve, so as to guarantee some flexibility in the sort of patterns each neuron may locally capture.
And how exactly will we shape our activation function? Well, one way to do this is by introducing a bias term into our model. This is depicted by the arrow leaving the first input node (marked with the number '1') in the following diagram:

Representatively, we can think of this bias as the weight of a fictional input. This fictional input is said to be always present, allowing our activation unit to fire at will, without requiring any input features to be explicitly present (as shown in the green circle previously). The motivation behind this term is to be able to manipulate the shape of our activation function, which in turn impacts the learning of our model. We want our shape to flexibly fit different patterns in our data. The weight of the bias term is updated in the same manner as all the other weights are. What makes it different is that it is not disturbed by its input neuron, which simply always holds a constant value (as shown previously).
So, how do we actually influence our activation threshold using this bias term? Well, lets consider a simplified example. Suppose we have some outputs generated by a stepped activation function, which produces either a '0'or a '1'for every output, like so:

We can then rewrite this formula to include the bias term, as follows:

In other words, we are using yet another mathematical trick and redefining the threshold value as the negative of our bias term (Threshold = -(bias)). This bias term is randomly initialized at the beginning of our training session, and is iteratively updated as the model sees more examples, and learns from these examples. Hence, it is important to understand that although we randomly initialize model parameters, such as the weights and biases, our hope is to actually show the model enough input examples and their corresponding output classes. In doing so, we want our model to learn from its errors, searching for the ideal parametric combinations of weights and bias corresponding to the correct output classes. Do note that when we initialize different weights, what we are actually doing is modifying the steepness of our activation function.
The following graph shows how different weights impact the steepness of a sigmoid activation function:

We essentially hope that by tinkering with the steepness of our activation function, we are able to ideally capture a certain underlying pattern in our data. Similarly, when we initialize different bias terms, what we are actually trying to do is shift the activation function in an optimal manner (to the left or to the right), so as to trigger activation corresponding to specific configurations of input and output features.
The following graph shows how different bias terms impact the position of a sigmoid activation function:
