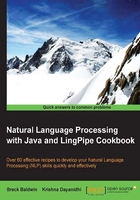
上QQ阅读APP看书,第一时间看更新
Understanding precision and recall
The false positive from the preceding recipe is one of the four possible error categories. All the categories and their interpretations are as follows:
- For a given category X:
- True positive: The classifier guessed X, and the true category is X
- False positive: The classifier guessed X, but the true category is a category that is different from X
- True negative: The classifier guessed a category that is different from X, and the true category is different from X
- False negative: The classifier guessed a category different from X, but the true category is X
With these definitions in hand, we can define the additional common evaluation metrics as follows:
- Precision for a category X is true positive / (false positive + true positive)
- The degenerate case is to make one very confident guess for 100 percent precision. This minimizes the false positives but will have a horrible recall.
- Recall or sensitivity for a category X is true positive / (false negative + true positive)
- The degenerate case is to guess all the data as belonging to category X for 100 percent recall. This minimizes false negatives but will have horrible precision.
- Specificity for a category X is true negative / (true negative + false positive)
- The degenerate case is to guess that all data is not in category X.
The degenerate cases are provided to make clear what the metric is focused on. There are metrics such as f-measure that balance precision and recall, but even then, there is no inclusion of true negatives, which can be highly informative. See the Javadoc at com.aliasi.classify.PrecisionRecallEvaluation
for more details on evaluation.
- In our experience, most business needs map to one of the three scenarios:
- High precision / high recall: The language ID needs to have both good coverage and good accuracy; otherwise, lots of stuff will go wrong. Fortunately, for distinct languages where a mistake will be costly (such as Japanese versus English or English versus Spanish), the LM classifiers perform quite well.
- High precision / usable recall: Most business use cases have this shape. For example, a search engine that automatically changes a query if it is misspelled better not make lots of mistakes. This means it looks pretty bad to change "Breck Baldwin" to "Brad Baldwin", but no one really notices if "Bradd Baldwin" is not corrected.
- High recall / usable precision: Intelligence analysis looking for a particular needle in a haystack will tolerate a lot of false positives in support of finding the intended target. This was an early lesson from our DARPA days.