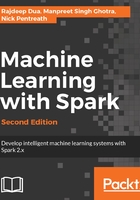
上QQ阅读APP看书,第一时间看更新
Vector operations
Vectors can be added together, subtracted, and multiplied by scalars. Other operations on vectors include finding the mean, normalization, comparison, and geometrical representation.
- The Add operation: This code shows an element-wise add operation on vector objects:
// vector's
val v1 = DenseVector(3, 7, 8.1, 4, 5)
val v2 = DenseVector(1, 9, 3, 2.3, 8)
// elementwise add operation
def add(): Unit = {
println(v1 + v2)
}
This last code gives us the result as follows: DenseVector(4.0, 16.0, 11.1, 6.3, 13.0)
- Multiply and Dot operation: It is an algebraic operation, which takes two sequences of an equal length of numbers, and returns a single number; algebraically, it is the sum of the products of the corresponding entries of the two sequences of numbers. It is mathematically represented as follows:

a b = |a| × |b| × cos(θ) OR a b = ax × bx + ay × by
import breeze.linalg.{DenseVector, SparseVector}
val a = DenseVector(0.56390, 0.36231, 0.14601, 0.60294,
0.14535)
val b = DenseVector(0.15951, 0.83671, 0.56002, 0.57797,
0.54450)
println(a.t * b)
println(a dot b)
This preceding code gives us the following result:
0.9024889161, 0.9024889161
import breeze.linalg.{DenseVector, SparseVector}
val sva =
SparseVector(0.56390,0.36231,0.14601,0.60294,0.14535)
val svb =
SparseVector(0.15951,0.83671,0.56002,0.57797,0.54450)
println(sva.t * svb)
println(sva dot svb)
The last code gives us the result as follows: 0.9024889161, 0.9024889161
- Finding the Mean: This operation returns the mean of the elements of the vector along the first array dimension, whose size does not equal 1. It is mathematically represented as follows:
import breeze.linalg.{DenseVector, SparseVector}
import breeze.stats.mean
val mean = mean(DenseVector(0.0,1.0,2.0))
println(mean)
This gives us the result as follows:
1.0
import breeze.linalg.{DenseVector, SparseVector}
import breeze.stats.mean
val svm = mean(SparseVector(0.0,1.0,2.0))
val svm1 = mean(SparseVector(0.0,3.0))
println(svm, svm1)
This gives us the result as follows:
(1.0,1.5)
- Normalized vector: Every vector has a magnitude, which is calculated using the Pythagoras theorem as |v| = sqrt(x^2 + y^2 + z^2); this magnitude is a length of a line from the origin point (0,0,0) to the point indicated by the vector. A vector is normal if its magnitude is 1. Normalizing a vector means changing it so that it points in the same direction (beginning from the origin), but its magnitude is one. Hence, a normalized vector is a vector in the same direction, but with norm (length) 1. It is denoted by ^X and is given by the following formula:

Where
is the norm of
. It is also called a unit vector.


import breeze.linalg.{norm, DenseVector, SparseVector}
import breeze.stats.mean
val v = DenseVector(-0.4326, -1.6656, 0.1253, 0.2877, -
1.1465)
val nm = norm(v, 1)
//Normalizes the argument such that its norm is 1.0
val nmlize = normalize(v)
// finally check if the norm of normalized vector is 1 or not
println(norm(nmlize))
This gives us the following result:
Norm(of dense vector) = 3.6577
Normalized vector is = DenseVector(-0.2068389122442966,
-0.7963728438143791, 0.05990965257561341, 0.1375579173663526,
-0.5481757117154094)
Norm(of normalized vector) = 0.9999999999999999
- Displaying the minimum and maximum element in a vector:
import breeze.linalg._
val v1 = DenseVector(2, 0, 3, 2, -1)
println(argmin(v1))
println(argmax(v1))
println(min(v1))
println(max(v1))
This gives us the result as follows:
4, 2, -1, 3
- Compare operation: This compares two vectors for equality and for less than, or greater than, operations:
import breeze.linalg._
val a1 = DenseVector(1, 2, 3)
val b1 = DenseVector(1, 4, 1)
println((a1 :== b1))
println((a1 :<= b1))
println((a1 :>= b1))
println((a1 :< b1))
println((a1 :> b1))
This gives us the following result:
BitVector(0), BitVector(0, 1), BitVector(0, 2),
BitVector(1),
BitVector(2)
- Geometrical representation of a vector:
