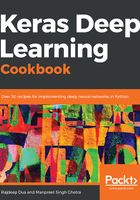
上QQ阅读APP看书,第一时间看更新
Create a Sequential model
We will create a Sequential network with four layers.
- Layer 1 is a dense layer which has input_shape of (*, 784) and an output_shape of (*, 32)
A dense layer is a regular densely-connected neural network layer. A Dense layer implements the operation output = activation(dot(input, kernel) + bias), where activation is the element-wise activation function passed as the activation argument, kernel is a weights matrix created by the layer, and bias is a bias vector created by the layer. (This is only applicable if use_bias is True).
- Layer 2 is an activation layer with the tanh Activation function applies activation to the incoming tensor:
keras.layers.Activation(activation)
Activation can also be applied as a parameter to the dense layer:
model.add(Dense(64, activation='tanh'))
- Layer 3 is a dense layer with output (*,10)
- Layer 4 has Activation that applies the softmax function:
Activation('softmax')
In mathematics, the softmax function, also called the normalized exponential function, is a generalization of the logistic function that squashes a K-dimensional vector z of arbitrary real values to a K-dimensional vector σ(z) of real values; each entry is in the range (0, 1), and all the entries add up to 1. The following formula shows this:
.

from keras.models import Sequential
from keras.layers import Dense, Activation
model = Sequential([
Dense(32, input_shape=(784,)),
Activation('tanh'),
Dense(10),
Activation('softmax'),
])
print(model.summary())
- The summary of the model created is printed in the following snippet:
Layer (type) Output Shape Param #
=================================================================
dense_1 (Dense) (None, 32) 25120
_________________________________________________________________
activation_1 (Activation) (None, 32) 0
_________________________________________________________________
dense_2 (Dense) (None, 10) 330
_________________________________________________________________
activation_2 (Activation) (None, 10) 0
=================================================================
Total params: 25,450
Trainable params: 25,450
Non-trainable params: 0
_______________________________________________________________
Sequential is a subclass of Model and has some additional methods, as shown in the following sections.