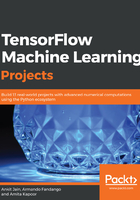
上QQ阅读APP看书,第一时间看更新
Multiple graphs
We can create our own graphs, which are separate from the default graph, and execute them in a session. However, creating and executing multiple graphs is not recommended, because of the following disadvantages:
- Creating and using multiple graphs in the same program would require multiple TensorFlow sessions, and each session would consume heavy resources
- Data cannot be directly passed in-between graphs
Hence, the recommended approach is to have multiple subgraphs in a single graph. In case we wish to use our own graph instead of the default graph, we can do so with the tf.graph() command. In the following example, we create our own graph, g, and execute it as the default graph:
g = tf.Graph()
output = 0
# Assume Linear Model y = w * x + b
with g.as_default():
# Define model parameters
w = tf.Variable([.3], tf.float32)
b = tf.Variable([-.3], tf.float32)
# Define model input and output
x = tf.placeholder(tf.float32)
y = w * x + b
with tf.Session(graph=g) as tfs:
# initialize and print the variable y
tf.global_variables_initializer().run()
output = tfs.run(y,{x:[1,2,3,4]})
print('output : ',output)
Now, let's put this learning into practice and implement the classification of handwritten digital images with TensorFlow.