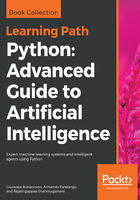
HMM parameter estimation
Now that we have defined both the forward and the backward algorithms, we can use them to estimate the structure of the underlying HMM. The procedure is an application of the Expectation-Maximization algorithm, which will be discussed in the next chapter, Chapter 5, EM Algorithm and Applications, and its goal can be summarized as defining how we want to estimate the values of A and B. If we define N(i, j) as the number of transitions from the state i to the state j, and N(i) the total number of transitions from the state i, we can approximate the transition probability P(i → j) with:

In the same way, if we define M(i, p) the number of times we have observed the emission op in the state i, we can approximate the emission probability P(op|xi) with:

Let's start with the estimation of the transition probability matrix A. If we consider the probability that the HMM is in the state i at time t, and in the state j at time t+1 given the observations, we have:

We can compute this probability using the forward and backward algorithms, given a sequence of observations o1, o2, ..., oSequence Length. In fact, we can use both the forward message fti, which is the probability that the HMM is in the state i after t observations, and the backward message bt+1j, which is the probability of a sequence ot+1, ot+1, ..., oSequence Length starting at time t+1, given that the HMM is in state j at time t+1. Of course, we need also to include the emission probability and the transition probability pij, which is what we are estimating. The algorithm, in fact, starts with a random hypothesis and iterates until the values of A become stable. The estimation αij at time t is equal to:

In this context, we are omitting the full proof due to its complexity; however, the reader can find it in A tutorial on hidden Markov models and selected applications in speech recognition, Rabiner L. R., Proceedings of the IEEE 77.2.
To compute the emission probabilities, it's easier to start with the probability of being in the state i at time t given the sequence of observations:

In this case, the computation is immediate, because we can multiply the forward and backward messages computed at the same time t and state i (remember that considering the observations, the backward message is conditioned to xt = i, while the forward message computes the probability of the observations joined with xt = i. Hence, the multiplication is the unnormalized probability of being in the state i at time t). Therefore, we have:

The proof of how the normalizing constant is obtained can be found in the aforementioned paper. We can now plug these expressions to the estimation of aij and bip:

In the numerator of the second formula, we adopted the indicator function (it's 1 only if the condition is true, 0 otherwise) to limit the sum only where those elements are ot = p. During an iteration k, pij is the estimated value aij found in the previous iteration k-1.
The algorithm is based on the following steps:
- Randomly initialize the matrices A and B
- Initialize a tolerance variable Tol (for example, Tol = 0.001)
- While Norm(Ak - Ak-1) > Tol and Norm(Bk - Bk-1) > Tol (k is the iteration index):
- For t=1 to Sequence Length-1:
- For i=1 to N:
- For j=1 to N:
- Compute αtij
- Compute βti
- For j=1 to N:
- For i=1 to N:
- Compute the estimations of aij and bip and store them in Ak
- For t=1 to Sequence Length-1:
Alternatively, it's possible to fix the number of iterations, even if the best solution is using both a tolerance and a maximum number of iterations, to terminate the process when the first condition is met.