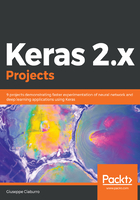
Supervised learning
In supervised learning, the algorithm generates a function that links input values to a desired output through the observation of a set of examples in which each data input has its relative output data, and that's used to construct predictive models. In this way, the machine can resolve the relevant tasks automatically. To do this, the input data is included in a set I, (typically vectors). Then, the set of output data is fixed as set O, and finally it defines a function f that associates each input with the correct answer. Such information is called a training set. The following diagram shows a generic supervised learning workflow:

As you can see, a series of images of fruits characterized by different shapes and colors are supplied as input. In the input data, the images are also classified into two categories (lemon and orange). In this way, the neural network will be able to classify each new image provided correctly .
All supervised learning algorithms are based on the following thesis: if an algorithm provides an adequate number of examples, it'll be able to create a derived function B that'll approximate the desired function A.
If the approximation of the desired function is adequate, when the input data is offered to the derived function, this function should be able to provide output responses similar to those provided by the desired function that are acceptable. These algorithms are based on the following concept: similar input values correspond to similar output values.
Generally, in the real world, this assumption isn't valid; however, some situations exist in which it's acceptable. Clearly, the proper functioning of such algorithms depends significantly on the input data. If there are only a few training input values, the algorithm might not have enough experience to provide a correct output. Conversely, many input values may make it excessively slow since the derivative function generated by a large number of inputs could be very complicated.
Moreover, experience shows that this type of algorithm is very sensitive to noise; even a few pieces of incorrect data can make the entire system unreliable and lead to wrong decisions. In supervised learning, it's possible to split problems based on the nature of the data. If the output value is categorical, such as membership/non-membership of a certain class, it's a classification problem. If the output is a continuous real value in a certain range, then it's a regression problem.