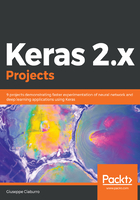
Data visualization
By visualizing the data, it is possible to understand the meaning of the data by positioning it in a visual context. A numerical analysis of data can hide at first sight patterns, that is, trends and correlations that instead represent the basis of data mining. These characteristics are highlighted through graphs and diagrams that describe the nature of the data under analysis.
The first thing we can do is plot a data plot. In this way, we will be able to highlight the characteristics we have already analyzed with the statistical data returned by the describe() function. A boxplot is a graphical representation that's used to describe the distribution of a sample by simple dispersion and position indexes. As we said in Chapter 2, Modeling Real Estate Using Regression Analysis, to plot a boxplot in Python, we can use the matplotlib library.
As always, let's start by importing the library into Python:
import matplotlib.pyplot as plt
The available data is in pandas Dataframe format. For this reason, we can use the pandas.DataFrame.boxplot function. This function makes a boxplot from DataFrame columns, which are optionally grouped by some other columns:
boxplot = data.boxplot(column=BHNames)
plt.show()
Finally, to print the output, the plt.show() function will be used. This function displays all figures and block until the figures have been closed. In the following diagram, the boxplots of all the variables contained in the Input DataFrame are shown:

The previous diagram highlights the results of data scaling. In fact, we can see that the mean of all the variables (triangle markers) is positioned on zero. Furthermore, we can note that some variables have outliers (circles out of the whiskers). Comparing the lengths of the two whiskers (which represent the distances between the 25th percentile and the minimum and between the 75th percentile and the maximum) and the heights of the two rectangles that make up the box (representing the distances between the 25th percentile and median (50th percentile) and between the median and the 75th percentile) information on the symmetry of the distribution is obtained: this is more symmetrical, as the lengths of the whiskers are similar to each other and the heights of the two rectangles are similar to one another.
Now we will look for possible relationships among the input variables. Relationships among variables can be highlighted through a suitable graphical representation, known as a scatter plot. A scatter plot helps us study the relationship between two quantitative variables (correlation), which are detected on the same units. Let's consider a Cartesian reference, where the values of a variable appear on the horizontal axis and those of the other variable on the vertical axis. Each point in the plot is specified by a pair of numerical coordinates that represent the values of the two variables that are detected at a specific observation.
A large number of points can be observed in a single dispersion diagram. More such points are placed around a straight line, that is, the greater the correlation between the two variables. If this straight line goes from the origin out to high x-y values, then it is said that the variables have a positive correlation. If the line moves from a high value on the Y axis to a high value on the X axis, the variables have a negative correlation, as shown in the following diagram:

By using scatter plots, we can gain an idea of the shape and strength of the relationship among the variables. Deviations due to abnormal data, which is specific values that deviate from the general scheme or presence of different clusters, can also be highlighted.
What happens when the pairs of variables to compare are numerous? In this case, we can use a scatter plot matrix. What is the scatter plot matrix? For a set of variables, A1, A2, .., Ak, the scatter plot matrix shows all the scatter plots of the variables in a matrix format. So, if there are n variables, the scatter plot matrix will have n rows and n columns, and the ith row and jth column of this matrix is a plot of Ai versus Aj.
To draw a scatter plot matrix, we will use the pandas.plotting.scatter_matrix() function, as follows:
pd.plotting.scatter_matrix(InputScaled, figsize=(6, 6))
plt.show()
The subplot in the ith row, jth column of the matrix is a scatter plot of the ith column of the matrix against the jth column of the matrix. Along the diagonal are histogram plots of each column of the matrix, as shown in the following diagram:

Analyzing the preceding diagram, no correlation seems to exist among the input variables, meaning that all of the variables are necessary for the correct classification of the target. To confirm this, we can perform a correlation analysis. In Python, correlation analysis is performed by the pandas.DataFrame.corr() function; it computes pairwise correlation of columns, excluding NA or null values, as follows:
CorData = InputScaled.corr(method='pearson')
with pd.option_context('display.max_rows', None,
'display.max_columns', CorData.shape[1]):
print(CorData)
The following results are returned:

All correlation coefficients are close to zero to indicate that no correlation exists. The variables are many, so an immediate control of this trend is not easy. To overcome this inconvenience, we can plot a correlogram. A correlogram is a graph of a correlation matrix. It is very useful to highlight the most correlated variables in a data table. In this plot, correlation coefficients are colored according to the value:
plt.matshow(CorData)
plt.xticks(range(len(CorData.columns)), CorData.columns)
plt.yticks(range(len(CorData.columns)), CorData.columns)
plt.colorbar()
plt.show()
The correlogram is shown in the following diagram:

The cells are all dark, and according to the heatmap legend, this means that there is no specific correlation among the data.