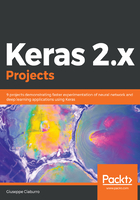
Gaussian mixture models
Mixture models are types of density models that are made up of a certain number of density functions, usually Gaussian. These functions are combined to provide multimode density. Mixed models allow you to represent probability distributions in the presence of subpopulations. For example, it can be used to model the colors of an object and exploit this information to perform color-based tracking or segmentation.
For the training of such models, the expectation maximization (EM) algorithm is used. This algorithm allows for learning with incomplete datasets or with datasets that have been generated by mixtures of probability distributions, that is, if neither of the parameters of the distribution functions is known, nor where each piece of data to a date distribution function belongs.
It is assumed that the patterns were generated from a mix of distributions: each class generated data according to a specific distribution, but at the end of the generation the patterns appear as products from a single multi-modal distribution. The goal of the algorithm is to trace-starting from the training set patterns-to the parameters of the individual distributions that generated them. To this end, the form of the distributions is assumed and it is assumed, for simplicity, that they are all of the same type. The most frequent case is that of mixing multinormal (Gaussian) distributions, whose definition parameters are to be estimated.