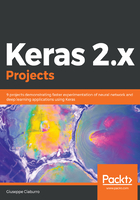
Summary
In this chapter, you've learned about the different types of regression techniques. Regression analysis is a statistical process that's done to study the relationship between a set of independent variables (explanatory variables) and a dependent variable (response variable). Regression algorithms show you how the value of the response variable changes when the explanatory variable is varied. The concepts underlying regression were explored. Furthermore, we gained an understanding of the differences between simple and multiple regression. Later, we saw how a simple and multiple linear regression problem is represented algebraically. Thus, we have analyzed how a regression problem is solved through the least squares algorithm.
The second part of this chapter was dedicated to the practical resolution of a multiple regression problem using the keras library. Modeling a real estate market is a typical regression problem that different operators in the sector face daily. We started with an exploratory analysis to understand the data at our disposal, performing visual analysis by tracing a barplot to identify possible trends. After that, we built a fully densely connected neural network to model this problem. Finally, to evaluate the performance of the model, the problem was addressed by constructing a multiple linear regression model: two models were then compared. The Keras model produced much better results.
In the next chapter, we will be introduced to the basic concepts of the classification problem. We will explore different types of classification techniques, understand the basic concepts of classification methods, and how to implement them in the Keras environment. We will also learn how to import and organize data for neural network classification analysis, learn how to implement a Keras classification model using a real-life example, and how to evaluate the model's performance. Finally, we will learn how to tune a model to improve its performance.