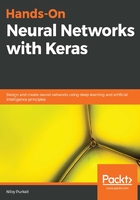
上QQ阅读APP看书,第一时间看更新
Compiling the model
Next, we will compile our Keras model. Compilation basically refers to the manner in which your neural network will learn. It lets you have hands-on control of implementing the learning process, which is done by using the compile method that's called on our model object. The method takes at least three arguments:
model.compile(optimizer='resprop', #'sgd'
loss='sparse_categorical_crossentropy',
metrics=['accuracy'])
Here, we describe the following functions:
- A loss function: This simply measures our performance on the training data, compared to the true output labels. Due to this, the loss function can be used as an indication of our model's errors. As we saw earlier, this metric is actually a function that determines how far our model's predictions are from the actual labels of the output classes. We saw the Mean Squared Error (MSE) loss function in Chapter 2, A Deeper Dive into Neural Networks, of which many variations exist. These loss functions are implemented in Keras, depending on the nature of our ML task. For example, if you wish to perform a binary classification (two output neurons representing two output classes), you are better off choosing binary cross-entropy. For more than two categories, you may try categorical cross-entropy, or sparse categorical cross-entropy. The former is used when your output labels are one-hot encoded, whereas the latter is used when your output classes are numerical categorical variables. For regression problems, we often advise the MSE loss function. When dealing with sequence data, as we will later, then Connectionist Temporal Classification (CTC) is deemed a more appropriate type of loss function. Other flavors of loss may differ in the manner they measure the distance between predictions and actual output labels (for example, cosine_proximity uses a cosine measure of distance), or the choice of probability distribution to model the predicted values (for example, the Poisson loss function is perhaps better if you are dealing with count data).
- An optimizer: An intuitive way to think of an optimizer is that it tells the network how to get to a global minimum loss. This includes the goal you want to optimize, as well as the size of the step it will take in the direction of your goal. Technically, the optimizer is often described as the mechanism that's employed by the network to self-update, which is does by using the data it is fed and the loss function. Optimization algorithms are used to update weights and biases that are the internal parameters of a model in the process of error reduction. There are actually two distinct types of optimization functions: functions with constant learning rates (such as Stochastic Gradient Decent (SGD)) and functions with adaptive learning rates (such as Adagrad, Adadelta, RMSprop, and Adam). The latter of the two are known for implementing heuristic-based and pre-parameterized learning rate methods. Consequentially, using adaptive learning rates can lead to less work in tuning the hyperparameters of your model.
- metrics: This simply denotes the evaluation benchmark we monitor during training and testing. Accuracy is most commonly used, but you may design and implement a custom metric through Keras, if you so choose. The main functional difference between the loss and accuracy score that's shown by the metric is that the accuracy measure is not involved in the training process at all, whereas loss is used directly in the training process by our optimizer to backpropagate the errors.