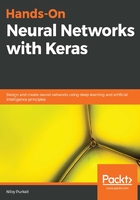
Experimenting with TensorFlow playground
Let's see how different neurons can actually capture different patterns in our data using a dummy example. Suppose that we have two output classes in our data, as plotted in the following diagram. The task of our neural network is to learn the decision boundaries separating our two output classes. Plotting this two-dimensional dataset, we get something similar to the following diagram, where we see several decision boundaries that classify the different possible outputs:

We will employ a phenomenal open-source tool to visualize our model's learnings, known as TensorFlow playground. This tool simply allows to simulate a neural network with some synthetic data, and lets us actually see what patterns our neurons are picking up on. It lets you tinker with all the concepts we have overviewed so far, including different types and forms of input features, activation functions, learning rate, and many more. We highly encourage you to experiment with the different synthetic datasets they provide, play with the input features and progressively add neurons, as well as hidden layers to see how this affects learning. Do also experiment with different activation functions to see how your model can capture various complex patterns from data. Seeing, is indeed believing! (Or more scientifically put, nullius in verba). As we can see in our diagram below, both neurons in the hidden layer are actually capturing different curvatures in our feature space, learning a specific pattern in the data. You can visualize the weights of our model by observing the thickness of the lines connecting the layers. You can also visualize the output of each neuron (the shaded blue and white areas shown within the neurons) to see what underlining pattern that specific neuron is capturing in our data. This representation, as you will see by experimenting on the playground, is iteratively updated and converges to an ideal value, given the form and type of the data, activation functions, and learning rates used:

