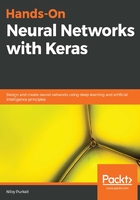
Backpropagation
For the more mathematically oriented, you must be wondering how exactly we descend our gradient iteratively. Well, as you know, we start by initializing random weights to our model, feed in some data, compute dot products, and pass it through our activation function along with our bias to get a predicted output. We use this predicted output and the actual output to estimate the errors in our model's representations, using the loss function. Now here comes the calculus. What we can do now is differentiate our loss function, J(θ), with respect to the weights of our model (θ). This process essentially lets us compare how changes in our model's weights affect the changes in our model's loss. The result of this differentiation gives us the gradient of our J(θ) function at the current model weight (θ) along with the direction of the highest ascent. By highest ascent, we meant the direction in which the difference between prediction and output values seem higher. Hence, we simply take a step in the opposite direction, and descend the gradient of our loss function, J(θ), with respect to our model weights (θ). We present an algorithmic representation of this concept, in form of pseudo-code, as follows:

The following graph is a visualization of the gradient descent algorithm:

As we see, the gradient descent algorithm allows us to take steps down the loss hyperplane, until our model converges to some optimal parameters. At this point, the difference between our model's predictions and reality will be quite negligible, and we can consider our model trained!:

Thus, we compute the changes in our network weights with respect to the changes of the values generated by the loss function (i.e. the gradients of the network weights). Then, we proportionally update the network weights, in the opposite direction of the computed gradients, so as to adjust for the errors.