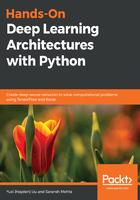
Who this book is for
If you're a data scientist, machine learning developer/engineer, deep learning practitioner, or are curious about the field of AI and want to upgrade your knowledge of various deep learning architectures, this book will appeal to you. You are expected to have some knowledge of statistics and machine learning algorithms to get the most out of this book.
About the authors
Yuxi (Hayden) Liu is an author of a series of machine learning books and an education enthusiast. His first book, the first edition of Python Machine Learning By Example, was a #1 bestseller on Amazon India in 2017 and 2018 and his other book R Deep Learning Projects, both published by Packt Publishing.
He is an experienced data scientist who is focused on developing machine learning and deep learning models and systems. He has worked in a variety of data-driven domains and has applied his machine learning expertise to computational advertising, recommendations, and network anomaly detection. He published five first-authored IEEE transaction and conference papers during his master's research at the University of Toronto.
Saransh Mehta has cross-domain experience of working with texts, images, and audio using deep learning. He has been building artificial, intelligence-based solutions, including a generative chatbot, an attendee-matching recommendation system, and audio keyword recognition systems for multiple start-ups. He is very familiar with the Python language, and has extensive knowledge of deep learning libraries such as TensorFlow and Keras. He has been in the top 10% of entrants to deep learning challenges hosted by Microsoft and Kaggle.
About the reviewers
Antonio L. Amadeu is a data science consultant and is passionate about data, artificial intelligence, and neural networks, in particular, using machine learning and deep learning algorithms in daily challenges to solve all types of issues in any business field and industry. He has worked for large companies, including Unilever, Lloyds Bank, TE Connectivity, and Microsoft.
As an aspiring astrophysicist, he does some research in relation to the Virtual Observatory group at Sao Paulo University in Brazil, a member of the International Virtual Observatory Alliance (IVOA).
Junho Kim received a BS in mathematics and computer science engineering in 2015, and an MS in computer science engineering in 2017, from Chung-Ang University, Seoul, South Korea. After graduation, he worked as an artificial intelligence research intern at AIRI, Lunit, and Naver Webtoon. Currently, he is working for NCSOFT as an artificial intelligence research scientist.
His research interests include deep learning in computer vision, especially in relation to generative models with GANs, and image-to-image translation. He likes to read papers and implement deep learning in a simple way that others can understand easily. All his works are shared on GitHub (@taki0112). His dream is to make everyone's life more fun using AI.
Packt is searching for authors like you
If you're interested in becoming an author for Packt, please visit authors.packtpub.com and apply today. We have worked with thousands of developers and tech professionals, just like you, to help them share their insight with the global tech community. You can make a general application, apply for a specific hot topic that we are recruiting an author for, or submit your own idea.
Preface
Deep learning architectures are composed of multilevel nonlinear operations that represent high-level abstractions. This allows you to learn useful feature representations from data. Hands-On Deep Learning Architectures with Python gives you a rundown explaining the essential learning algorithms used for deep and shallow architectures. Packed with practical implementations and ideas to build efficient artificial intelligence systems, this book will help you learn how neural networks play a major role in building deep architectures.
You will gain an understanding of various deep learning architectures, such as AlexNet, VGG Net, GoogleNet, and many more, with easy-to-follow code and diagrams. In addition to this, the book will also guide you in building and training various deep architectures, such as the Boltzmann mechanism, autoencoders, convolutional neural networks (CNNs), recurrent neural networks (RNN), natural language processing (NLP), generative adversarial networks (GANs), and others, with practical implementations. This book explains the essential learning algorithms used for deep and shallow architectures.
By the end of this book, you will be able to construct deep models using popular frameworks and datasets with the required design patterns for each architecture. You will be ready to explore the possibilities of deep architectures in today's world.