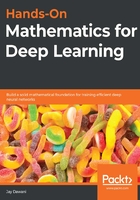
Conditional probability
Conditional probabilities are useful when the occurrence of one event leads to the occurrence of another. If we have two events, A and B, where B has occurred and we want to find the probability of A occurring, we write this as follows:

Here, .
However, if the two events, A and B, are independent, then we have the following:

Additionally, if , then it is said that B attracts A. However, if A attracts BC, then it repels B.
The following are some of the axioms of conditional probability:
.
.
.
is a probability function that works only for subsets of B.
.
- If
, then
.
The following equation is known as Bayes' rule:

This can also be written as follows:

Here, we have the following:
is called the prior.
is the posterior.
is the likelihood.
acts as a normalizing constant.

Often, we end up having to deal with complex events, and to effectively navigate them, we need to decompose them into simpler events.
This leads us to the concept of partitions. A partition is defined as a collection of events that together makes up the sample space, such that, for all cases of Bi, .
In the coin flipping example, the sample space is partitioned into two possible events—heads and tails.
If A is an event and Bi is a partition of Ω, then we have the following:

We can also rewrite Bayes' formula with partitions so that we have the following:

Here, .