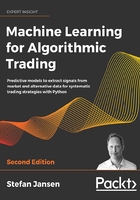
The market for alternative data
The investment industry spent an estimated $2-3 billion on data services in 2018, and this number is expected to grow at a double-digit rate per year in line with other industries. This expenditure includes the acquisition of alternative data, investments in related technology, and the hiring of qualified talent.
A survey by Ernst & Young shows significant adoption of alternative data in 2017; 43 percent of funds were using scraped web data, for instance, and almost 30 percent were experimenting with satellite data (see Figure 3.1). Based on the experience so far, fund managers considered scraped web data and credit card data to be most insightful, in contrast to geolocation and satellite data, which around 25 percent considered to be less informative:

Figure 3.1: Usefulness and usage of alternative data (Source: Ernst & Young, 2017)
Reflecting the rapid growth of this new industry, the market for alternative data providers is quite fragmented. J.P. Morgan lists over 500 specialized data firms, while AlternativeData.org lists over 300. Providers play numerous roles, including intermediaries such as consultants, aggregators, and tech solutions; sell-side supports deliver data in various formats, ranging from raw to semi-processed data or some form of a signal extracted from one or more sources.
We will highlight the size of the main categories and profile a few prominent examples to illustrate their persity.
Data providers and use cases
AlternativeData.org (supported by the provider YipitData) lists several categories that can serve as a rough proxy for activity in various data-provider segments. Social sentiment analysis is by far the largest category, while satellite and geolocation data have been growing rapidly in recent years:

The following brief examples aim to illustrate the broad range of service providers and potential use cases.
Social sentiment data
Social sentiment analysis is most closely associated with Twitter data. Gnip was an early social-media aggregator that provided data from numerous sites using an API and was acquired by Twitter in 2014 for $134 million. Search engines are another source that became prominent when researchers published, in Nature, that investment strategies based on Google Trends for terms such as debt could be used for a profitable trading strategy over an extended period (Preis, Moat, and Stanley 2013).
Dataminr
Dataminr was founded in 2009 and provides social-sentiment and news analysis based on an exclusive agreement with Twitter. The company is one of the larger alternative providers and raised an additional $391 million in funding in June 2018, led by Fidelity, at a $1.6 billion valuation, bringing total funding to $569 billion. It emphasizes real-time signals extracted from social media feeds using machine learning and serves a wide range of clients, including not only buy - and sell-side investment firms, but also news organizations and the public sector.
StockTwits
StockTwits is a social network and micro-blogging platform where several hundred thousand investment professionals share information and trading ideas in the form of StockTwits. These are viewed by a large audience across the financial web and social media platforms. This data can be exploited because it may reflect investor sentiment or itself drive trades that, in turn, impact prices. Nasseri, Tucker, and de Cesare (2015) built a trading strategy on selected features.
RavenPack
RavenPack analyzes a large amount of perse, unstructured, text-based data to produce structured indicators, including sentiment scores, that aim to deliver information relevant to investors. The underlying data sources range from premium newswires and regulatory information to press releases and over 19,000 web publications. J.P. Morgan tested a long-short sovereign bond and equity strategies based on sentiment scores and achieved positive results, with a low correlation to conventional risk premiums (Kolanovic and Krishnamachari, 2017).
Satellite data
RS Metrics, founded in 2010, triangulates geospatial data from satellites, drones, and airplanes with a focus on metals and commodities, as well as real estate and industrial applications. The company offers signals, predictive analytics, alerts, and end-user applications based on its own high-resolution satellites. Use cases include the estimation of retail traffic at certain chains or commercial real estate, as well as the production and storage of certain common metals or employment at related production locations.
Geolocation data
Advan, founded in 2015, serves hedge fund clients with signals derived from mobile phone traffic data, targeting 1,600 tickers across various sectors in the US and EU. The company collects data using apps that install geolocation codes on smartphones with explicit user consent and track location using several channels (such as Wi-Fi, Bluetooth, and cellular signal) for enhanced accuracy. The use cases include estimates of customer traffic at physical store locations, which, in turn, can be used as input to models that predict the top-line revenues of traded companies.
Email receipt data
Eagle Alpha provides, among other services, data on a large set of online transactions using email receipts, covering over 5,000 retailers, including SKU-level transaction data categorized into 53 product groups. J.P. Morgan analyzed a time series dataset, covering 2013-16, that covered a constant group of users active throughout the entire sample period. The dataset contained the total aggregate spend, number of orders, and number of unique buyers per period (Kolanovic and Krishnamachari, 2017).